A Novel Six Metastasis-Related Prognostic Gene Signature for Patients With Osteosarcoma
- Department of Orthopedics, Renmin Hospital of Wuhan University, Wuhan, China
Osteosarcoma is the most common malignant bone tumor, and although there has been significant progress in its management, metastases often herald incurable disease. Here we defined genes differentially expressed between primary and metastatic osteosarcoma as metastasis-related genes (MRGs) and used them to construct a novel six-MRG prognostic signature for overall survival of patients with osteosarcoma. Validation in internal and external datasets confirmed satisfactory accuracy and generalizability of the prognostic model, and a nomogram based on the signature and clinical variables was constructed to aid clinical decision-making. Of the six MRGs, FHIT is a well-documented tumor suppressor gene that is poorly defined in osteosarcoma. Consistent with tumor suppressor function, FHIT was downregulated in osteosarcoma cells and human osteosarcoma samples. FHIT overexpression inhibited osteosarcoma proliferation, migration, and invasion both in vitro and in vivo. Mechanistically, FHIT overexpression upregulate the epithelial marker E-cadherin while repressing the mesenchymal markers N-cadherin and vimentin. Our six-MRG signature represents a novel and clinically useful prognostic biomarker for patients with osteosarcoma, and FHIT might represent a therapeutic target by reversing epithelial to mesenchymal transition.
Introduction
Osteosarcoma is the most common primary malignant bone tumor. It mainly occurs in children and adolescents with an incidence of approximately one to four cases per million each year (Kansara et al., 2014; Gianferante et al., 2017; Whelan and Davis, 2018). Osteosarcoma usually arises from mesenchymal stem cells in the metaphyses of long bones such as the distal femur, proximal tibia, and proximal humerus (Ritter and Bielack, 2010; Deng et al., 2019). Osteosarcoma is highly aggressive and consequently incurs significant morbidity and mortality. There has been significant progress in the diagnosis and treatment of osteosarcoma over the last thirty years, which has positively impacted survival. The current standard of care for osteosarcoma is neoadjuvant chemotherapy with doxorubicin, cisplatin, and methotrexate combined with surgical resection (Prudowsky and Yustein, 2020; Marchandet et al., 2021), which, for early stage patients, has increased 5-year survival rates from 10 to 60–70% (Whelan and Davis, 2018). However, patients with relapsed and metastatic osteosarcoma—the main cause of death in osteosarcoma patients—still represent a management challenge, and their 5-year survival has remained unchanged at ∼15–30% (Kansara et al., 2014; Lin et al., 2017). Unfortunately, approximately 10–20% of osteosarcoma patients have detectable metastases at diagnosis, with the lung is the most common site of metastasis (Harrison et al., 2018). Determining the natural history and prognosis of osteosarcoma patients remains difficult due to a lack of reliable biomarkers, and novel strategies and reliable prognostic markers for osteosarcoma are urgently required. Determining the molecular mechanisms underlying osteosarcoma metastasis and identifying novel therapeutic targets or biomarkers would provide novel strategies for the treatment and prognostication of patients with osteosarcoma.
Over the last decade, high-throughput sequencing has revolutionized cancer genetics and provided comprehensive molecular portraits of osteosarcoma evolution (Gianferante et al., 2017; Rickel et al., 2017; Sayles et al., 2019; Zhao et al., 2020). Through the analysis of high-throughput sequencing data, a number of genetic and epigenetic abnormalities have been discovered and experimentally confirmed to be associated with osteosarcoma progression and may therefore be good prognostic biomarkers (Wu et al., 2020; Zhou et al., 2020). For example, in their analysis of TCGA data, Wu et al. (2020) reported that high expression of FGD1 was associated with poor prognosis in osteosarcoma patients and confirmed higher protein expression of FGD1 in osteosarcoma tissues than in adjacent normal tissues. Functionally, aberrant FGD1 expression might promote tumor cell proliferation and invasion by activating PI3K/AKT signaling. Liu et al. (2019) constructed a two-gene signature using seed genes (coefficient of variation larger than 20%) identified in gene expression data from the Gene Expression Omnibus (GEO) database and confirmed it as an independent prognostic marker in osteosarcoma. However, the full repertoire of genes driving osteosarcoma progression is unknown.
Motivated by these previous efforts and a need for a prognostic biomarker, here we analyzed the expression profiles of osteosarcoma samples obtained from the GEO database and identified genes that were differentially expressed (DEGs) between primary and metastatic osteosarcoma samples. We hypothesized that these DEGs were associated with metastasis and so defined them as metastasis-related genes (MRGs), which we subjected to functional analysis. A prognostic model based on six MRGs was constructed and validated in the internal TCGA osteosarcoma cohort and external GSE39055 cohort. Of the six MRGs, FHIT was a potent tumor suppressor that was downregulated in osteosarcoma cell lines and tissue samples. The effects of FHIT on osteosarcoma cell proliferation, migration, and invasion were examined in vitro and in vivo and the mechanism of action of FHIT in osteosarcoma metastasis further explored.
Materials and Methods
Data Collection
The gene matrices of 84 osteosarcoma samples and corresponding clinical information were downloaded from the TARGET database.1 Samples without follow-up were excluded to produce a final dataset of 81 osteosarcoma samples (TCGA cohort) for subsequent analysis. The GSE39055 cohort containing 37 osteosarcoma samples with clinical follow-up was downloaded from the National Center for Biotechnology Information (NCBI) GEO repository.2 The clinicopathological characteristics of the TCGA and GSE39055 cohorts are shown in Table 1. GSE14359 and GSE32981 datasets containing primary and metastatic osteosarcoma were download from the GEO database, with GSE14359 containing 10 primary OS samples and 8 metastatic OS samples and GSE32981 containing 12 primary OS samples and 11 metastatic OS samples.
Differential Analysis
Differentially expressed genes (DEGs) between primary and metastatic osteosarcoma samples were identified using R software and limma package. DEGs were identified with a cutoff of | log2 (fold change)| > 0.5 and P < 0.05. Shared DEGs in the GSE14359 dataset and GSE32981 dataset were defined as MRGs.
Functional Analysis
We conducted GO (gene ontology) and KEGG (Kyoto Encyclopedia of Genes and Genomes) pathway enrichment analysis on 160 identified MRGs and DEGs between high- and low-risk groups using DAVID (Database for Annotation, Visualization, and Integrated Discovery). A P-value < 0.05 was regarded as significant enrichment. Gene set enrichment analysis (GSEA) software was used to interrogate the gene expression matrices of the TCGA and GSE39055 cohorts.
PPI Network Construction
MRGs were used to construct a protein-protein interaction (PPI) network using the STRING database.3 Cytoscape software was used to reconstruct and visualize the network. Hub genes were identified using the cytohubba plugin. The number of adjacent nodes of the MRGs were visualized in R.
Construction and Validation of the MRG-Based Prognostic Signature
Details of the construction of the MRG-based prognostic signature are shown in Figure 1. Briefly, the TCGA cohort was randomly divided into a training cohort and a validation cohort in an approximately 1:1 ratio. Univariate Cox proportional hazard regression analysis was performed to detected prognostic genes. Then, Lasso (least absolute shrinkage and selector operator) regression analysis was carried out to identify the most powerful prognostic genes using the glmnet package in R, followed by stepwise multivariate Cox regression to construct the MRG-based prognostic signature. The risk score was calculated using the following formula: Risk score = (Exp = expression level; Coe = regression coefficient). Patients in the training cohort, validation cohort, entire cohort, and external GSE39055 cohort were divided into low- and high-risk groups based on the median risk score value in the training cohort. Kaplan-Meier (KM) survival analysis was performed to assess differences in overall survival between the low- and high-risk groups using the survival package in R. Time-dependent receiver operating characteristic (ROC) curve analysis was carried out to compute the sensitivity and specificity of the six-MRG signature in predicting the survival of osteosarcoma patients using the survivalROC package in R.
Tissue Collection
Twenty-four frozen tissue samples, including 12 osteosarcoma tissues and 12 matched adjacent normal tissues, were collected from patients with a histopathological diagnosis of osteosarcoma undergoing surgery in Renmin Hospital of Wuhan University between June 2018 and July 2020. These samples were harvested immediately after surgical resection and stored in liquid nitrogen until RNA or protein extraction. All patients signed informed consent, and the research the Research Ethics Committee of Renmin Hospital of Wuhan University approved the study protocol.
Osteosarcoma Cell Lines and Lentivirus Infection
The human osteoblast cell line hFOB1.19 and human osteosarcoma cell lines 143B, MG63, U2OS, and HOS were purchased from the Cell Bank of Type Culture Collection (CBTCC, Chinese Academy of Sciences, Shanghai, China) and Cell Bank of Wuhan University (Wuhan, China). hFOB1.19 cells were cultured in DMEM/F-12 complete medium. U2OS cells were cultured in McCoy’s 5a complete medium, while MG63 and 143B cells were cultured in α-MEM complete medium. All complete media were supplemented with 10% FBS (Gibco, Thermo Fisher Scientific, Waltham, MA, United States) and 1% antibiotics (100 units/mL penicillin and 100 units/mL streptomycin). All cells were maintained in a humidified incubator at 37°C and 5% CO2 atmosphere. To construct stable osteosarcoma cell lines overexpressing FHIT, lentivirus containing pLVX-FHIT-Puro and pLVX-Puro (vector) were purchased from Genechem (GeneChem, Shanghai, China) and were used to infect 143B and MG63 cells according to the manufacturer’s protocol. Stably transfected osteosarcoma cells were selected using puromycin (1.5 μg/mL) and confirmed by western blotting and qRT-PCR.
CCK-8 and Colony Formation Assays
CCK-8 (Cell Counting Kit-8) and colony formation assays were carried out to assess osteosarcoma cell proliferation. For the CCK-8 assay, FHIT-overexpressing and control 143B and MG63 cells were seeded in 96-well plates in triplicate at a density of 104 cells/well. The CCK-8 reagent (Dojindo Laboratories Co., Ltd., Kumamoto, Japan) was added to wells at the indicated timepoints. The plate was incubated for 1 h at 37°C before reading the optical density (OD) at 450 nm. For the colony formation assay, stably transfected 143B and MG63 cells were harvested and seeded into six-well plates at a density of 5 × 102 cells/well. Cells were cultured for 2 weeks, with the medium replaced every 3 days. After 2 weeks, colonies were fixed and stained with 1% crystal violet. The plates were photographed, and the number of colonies in every well was counted.
Wound Healing and Transwell Invasion Assays
Wound healing and transwell invasion assays were performed to evaluate the migration and invasion of osteosarcoma cells. For the wound healing assay, transfected osteosarcoma cells were seeded in six-well plates. When the cell density reached approximately 90%, cells were scratched with a 200 μL sterile pipette tip to create a gap and cultured in serum-free medium for 48 h. The gaps were observed and photographed at 0 and 48 h by inverted microscopy (Olympus, Tokyo, Japan). Matrigel chambers (Corning Costar, Corning, NY, United States) were used for the transwell invasion assay. Briefly, transfected osteosarcoma cells were digested and re-suspended in serum-free medium at a density of 106 cells/mL. Hundred microliter of cell suspension was added into the upper chamber, and 600 μL of medium (20% FBS) was added into the sub-chamber. 48 h later, cells in the bottom chamber were fixed and stained with 1% crystal violet before being photographed by inverted microscopy. The number of migrating cells was analyzed by ImagePro Plus 5.0 (Media Cybernetics, Rockville, MD, United States).
RNA Isolation and qRT-PCR
Total RNA from osteosarcoma samples and cells was isolated using TRIzol reagent (Invitrogen, Carlsbad, CA, United States) according to the manufacturer’s protocol. One microgram total RNA was reverse transcribed into cDNA using the RevertAid First Strand cDNA Synthesis Kit (Thermo Fisher Scientific). Then, qRT-PCR was conducted using the SYBR Green Mix (Vazyme, China). Relative gene expression was calculated by the 2–ΔΔCt method, and GAPDH expression served as an internal control. The primer sequences for qRT-PCR are listed as follow: GAPDH, forward, 5′-CTGAGTACGTCGTGGAGTCC-3′ and reverse, 5′-GTCTTCTGGGTGGCAGTGAT-3′; FHIT, forward, 5′-GCCAACATCTCATCA-AGCCC-3′ and reverse, 5′-AATCG GCCACTTCATCAGGA-3′; E-cadherin, forward, 5′-ACGCAT- TGCCACATACACTC-3′ and reverse, 5′-GGTGTTCACATC ATCGTCCG-3′; N-cadherin, forward, 5′-CCATCATTGCCATC CTGCTC-3′ and reverse, 5′-ATCAGGCTCCACAGTGTCAG-3′; vimentin, forward, 5′-GAGTCCACTGAGTACCGGAG-3′ and reverse, 5′-ACGAGCCATTTCCT-CCTTCA-3′
Western Blotting
Briefly, proteins were extracted from osteosarcoma samples and cells using RIPA lysis buffer (Cell Signaling Technology, Danvers, MA, United States), and the protein concentration was determined using the BCA protein assay. Proteins were separated by 10% SDS-PAGE gel electrophoresis and then transferred onto PVDF membranes. After blocking with 5% skimmed milk, membranes were incubated with specific antibodies at 4°C overnight. The next day, membranes were washed twice with TBST and incubated with horseradish peroxidase-linked secondary antibodies (1:10,000) at room temperature for 1 h. Blots were visualized using the ECL Kit (Servicebio, Wuhan, China) and analyzed with ImageJ software. Antibodies targeting GAPDH, Ki67, E-cadherin, N-cadherin, and vimentin were purchased from Cell Signaling Technology, and antibodies targeting FHIT were purchased from Abcam (Cambridge, United Kingdom).
Xenograft Tumor Model
Four-week-old male BALB/c nude mice were purchased from the Beijing HFK Experiment Animal Center (Beijing, China) and housed in the Animal Center of Wuhan University Renmin Hospital. Nude mice were injected subcutaneously with 5 × 106 stably transfected 143B cells or control cells. Tumor volumes were monitored using calipers every 7 days, and tumor volume was calculated as follows: Volume (mm3) = (length × width2)/2. Five weeks after cell injection, all mice were sacrificed and the xenografts weighed and harvested. All animal experiments were approved by the Animal Care and Use Committee of Wuhan University Renmin Hospital.
Immunohistochemistry Assay
Subcutaneous tumor tissues were fixed with 4% paraformaldehyde and embedded in paraffin. Then, 4 μm-thick tissue sections were stained with primary antibodies against target antigens (Ki67, E-cadherin, N-cadherin, and vimentin, all from Cell Signaling Technology) and a horseradish peroxidase-conjugated IgG (Servicebio). Proteins were visualized with DAB (3,3-diaminobenzidine), and the sections were mounted using transparent xylene. Positive brown staining was observed and photographed by light microscopy.
Statistical Analysis
All statistical analyses were conducted using R software (3.6.1) and GraphPad Prism version 8.0 software (GraphPad Software, La Jolla, CA, United States). All experiments were performed at least three times independently, and results are presented as means ± standard deviation (SD). Student’s t-test was used to analyze differences between two groups and one-way ANOVA was used to compare more than two groups. A P-value < 0.05 was regarded as statistically significant.
Results
Identification of Metastasis-Related Genes and Functional Enrichment Analysis
The flowchart of the study is presented in Figure 1. First, two osteosarcoma datasets were identified in the GEO database, both of which contained primary and metastatic osteosarcoma samples. There were 1448 upregulated and 1252 downregulated DEGs in GSE14359 and 348 upregulated and 460 downregulated DEGs in GSE32981 (Figures 2A,B). Of these, 160 DEGs were common to both datasets, so we defined these shared DEGs as MRGs (Figure 2C).
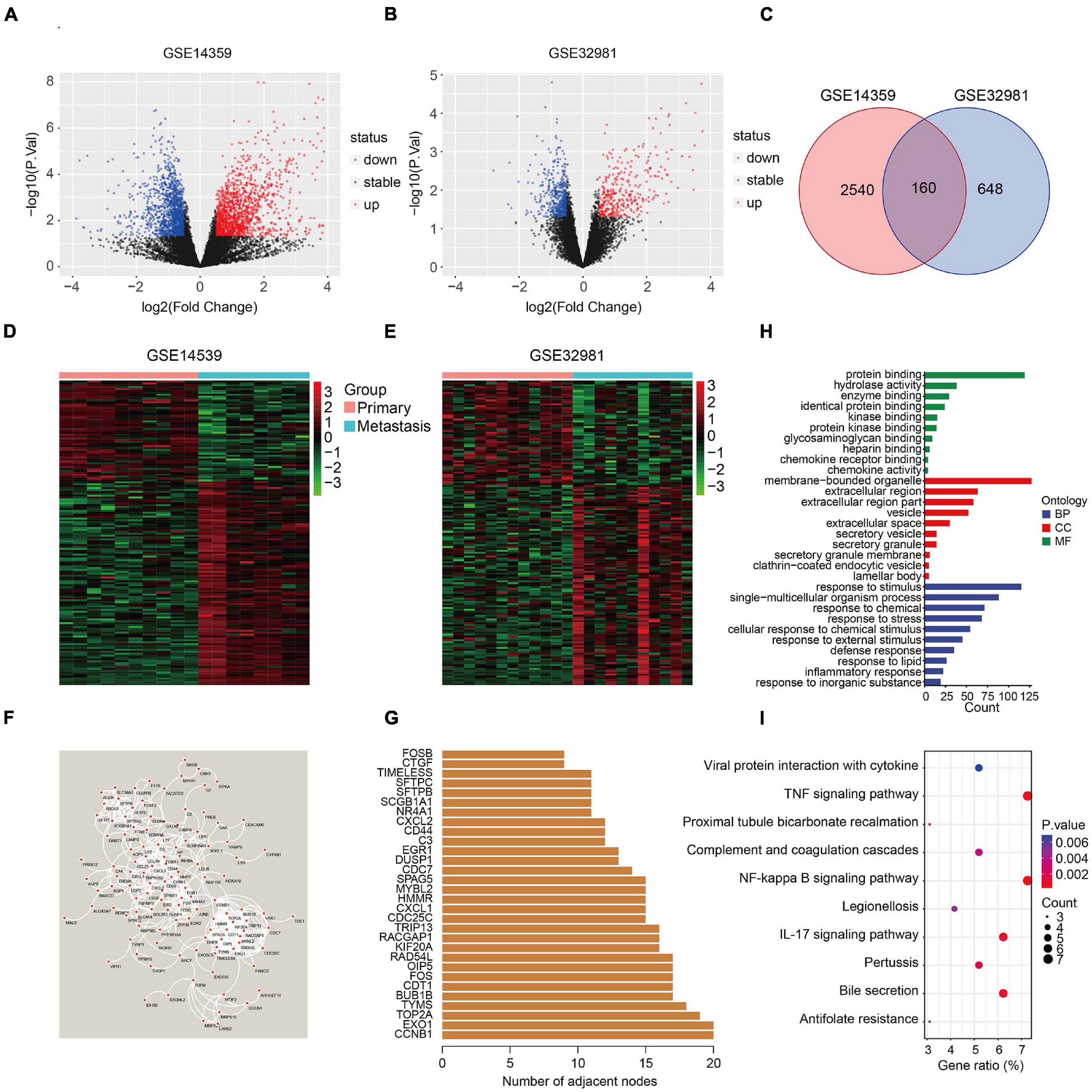
Figure 2. Identification of metastasis-related genes and functional enrichment analysis. (A,B) Volcano plot showing differentially expressed genes between primary and metastatic osteosarcoma. (C) Venn diagram of metastasis-related genes in osteosarcoma. (D,E) Heatmaps of metastasis-related genes in the GSE14539 and GSE32981 datasets. (F) Construction of a protein-protein interaction network using the metastasis-related genes. (G) The number of adjacent nodes of the metastasis-related genes. (H) GO analysis of the metastasis-related genes. (I) KEGG pathway enrichment analysis of the metastasis-related genes.
The expression of the 160 MRGs in the two GEO datasets is shown in Figures 2D,E and a protein-protein interaction (PPI) network for the 160 MRGs in Figure 2F. Hub gene analysis indicated that CCNB1 and EXO1 were the top two ranked genes in the PPI network (Figure 2G). GO analysis showed that response to stimulus, response to stress, response to external stimulus, defense response, and inflammatory response were significantly enriched processes in the 160 MRGs. In the cellular component (CC), MRGs were particularly enriched for membrane-bound organelles, extracellular regions, vesicles, extracellular space, and secretory vesicles. In terms of molecular function (MF), protein binding, hydrolase activity, and enzyme binding were the three most enriched terms (Figure 2H). KEGG pathway enrichment analysis revealed that MRGs primarily participated in the TNF and NF-kappa B signaling pathways (Figure 2I), two signaling pathways known drive metastasis in other cancers.
Construction of a Six-MRG Prognostic Model in the TCGA Osteosarcoma Cohort
To construct a prognostic model using the MRGs, the TCGA osteosarcoma cohort was randomly divided into a training cohort and a validation cohort at a ratio of approximately 1:1. In the training cohort, univariate Cox and Lasso regression analyses were conducted to determine the most powerful prognostic markers of the 160 MRGs (Figures 3A,B). Then, stepwise multivariate Cox regression analysis was performed to ultimately construct a six-MRG prognostic signature for osteosarcoma patients. The six MRGs were ABCA3, CTGF, AMIGO2, PREB, FHIT, and EXOSC5. The regression coefficients of the six MRGs are shown in Table 2, and the expression of the six MRGs in the training cohort are shown in Figure 3E.
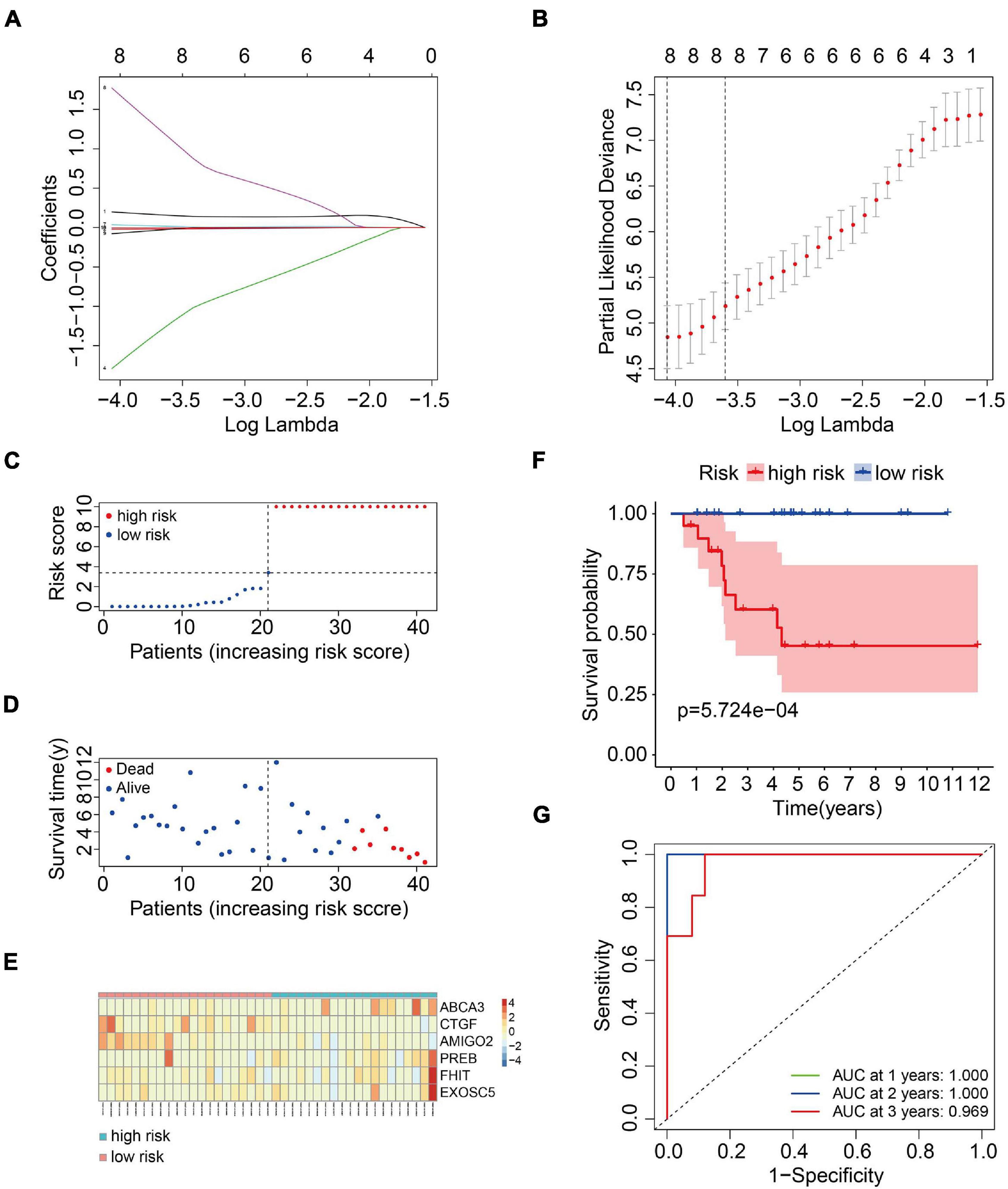
Figure 3. Construction of a six-MRG prognostic model in the training cohort. (A,B) Lasso regression and stepwise multivariate Cox regression were used to construct the six-MRG signature. (C) The risk score distribution of patients in the training cohort. (D) The survival status of patients in the training cohort. (E) mRNA expression profiles of the six MRGs in the training cohort. (F) Kaplan-Meier survival analysis of patients in the training cohort. (G) ROC curve analysis of the six-MRG signature in the training cohort.
Next, a risk score was calculated for each patient in the training cohort as follows: Risk score = (ABCA3 × 0.566 + CTGF × –0.057 + AMIGO2 × –5.564 + PREB × 0.175 + FHIT × 6.429 + EXOSC5 × –0.409). Taking the median value of the risk score as the cutpoint, patients in the training cohort were separated into low- and high-risk groups (Figure 3C). As shown in Figures 3D,E, patients in the high-risk group tended to have a shorter survival time and higher risk of death. Kaplan-Meier survival curve analysis showed that patients in high-risk group had significantly poorer survival than patients in the low-risk group (Figure 3F). To evaluate the predictive power of the six-MRG signature, time-dependent ROC curve analysis was carried out. As shown in Figure 3G, the area under the curve (AUC) was 1.00 at 1 year, 1.00 at 2 years, and 0.969 as 3 years, suggesting that the six-MRG signature effectively determined the prognosis of osteosarcoma patients.
Validation of the Six-MRG Prognostic Model in Internal and External Cohorts
We used an internal validation cohort, the entire cohort, and an external cohort to assess the reliability and stability of the six-MRG signature for prognostication. Using the same formula as above, the risk scores were calculated for patients in these cohorts. Then, patients were stratified into high- and low-risk groups according to the previously established median value in the training cohort. The risk score distribution of patients in the three cohorts are shown in Figures 4A–C, survival in Figures 4D–F, and the expression levels of the six MRGs in Figures 4G–I. Kaplan-Meier survival curve analysis confirmed that patients in the high-risk group had consistently and significantly poorer overall survival than patients in the low-risk group in all three cohorts (Figures 4J–L). The predictive power of the six-MRG signature was also evaluated using ROC curve analysis in the internal and external cohorts. In the validation cohort, the 1-, 2-, and 3-years overall survival AUCs of the six-MRG signature were 0.750, 0.824, and 0.725, respectively (Figure 4M). In the TCGA entire cohort, the 1-, 2-, and 3-years overall survival AUCs were 0.840, 0.869, and 0.805, respectively (Figure 4N). Furthermore, in the GSE39055 external cohort, the 1-, 2-, and 3-years overall survival AUCs were 0.938, 0.823, and 0.816, respectively (Figure 4O). Taken together, these validation results demonstrate satisfactory accuracy and generalizability of the prognostic model based on the six-MRG signature in osteosarcoma patients.
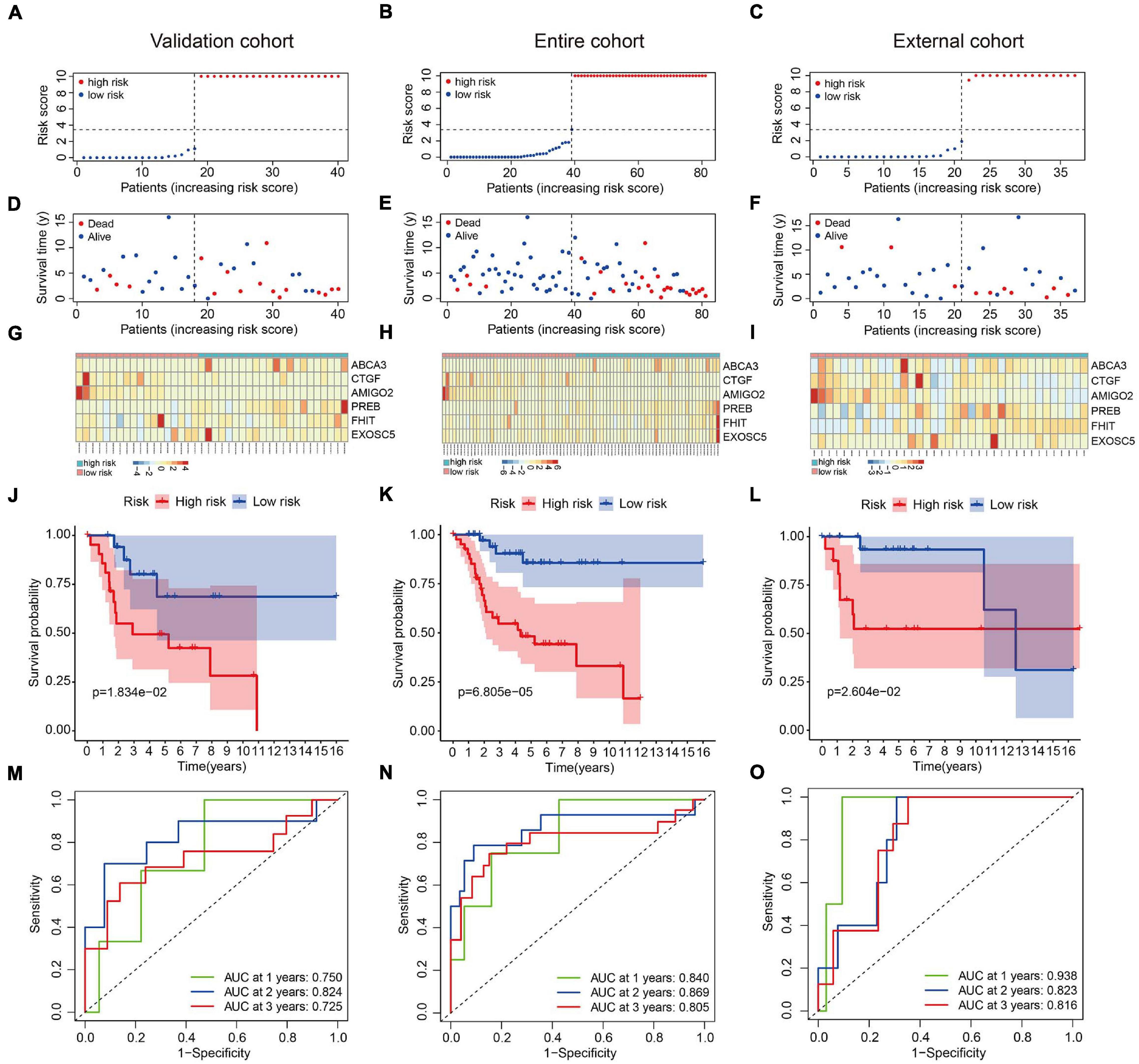
Figure 4. Validation of the six-MRG prognostic model in the internal and external cohorts. (A–C) The risk score distribution of patients in the validation cohort, entire cohort, and external cohort. (D–F) The survival status of osteosarcoma patients in the three cohorts. (G–I) The mRNA expression profiles of the six MRGs in the three cohorts. (J–L) Kaplan-Meier survival analysis of patients in the three cohorts. (M–O) ROC curve analysis of the six-MRG signature in the three cohorts.
The Six-MRG Signature Is Independent of Clinical Factors and Associated With Poor Prognosis and Adverse Clinical Features
In order to assess whether the six-MRG signature was independent of other clinical factors including gender, age, and tumor site, univariate and multivariate Cox regression analyses were performed in the TCGA osteosarcoma cohort (Table 3). The risk score was an independent prognostic marker in patients with osteosarcoma. We further examined the predictive value of the six-MRG signature in predicting outcomes in patients with different clinical features. Patients in the TCGA osteosarcoma cohort were divided into various clinical sub-groups including female and male and aged ≤ 15 and > 15 years before survival analysis according to high- and low-risk groups. Kaplan-Meier survival curve analysis showed that patients in the high-risk group had poorer overall survival than those in low-risk group in all clinical sub-groups (Figure 5).
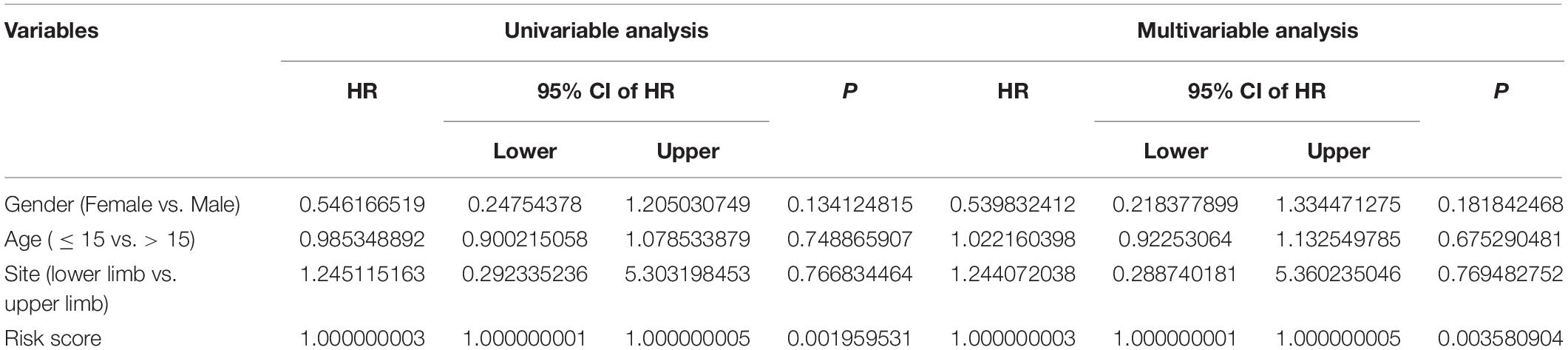
Table 3. Univariable and multivariable analysis of the six-MRG signature and clinical factors in the TCGA osteosarcoma cohort.
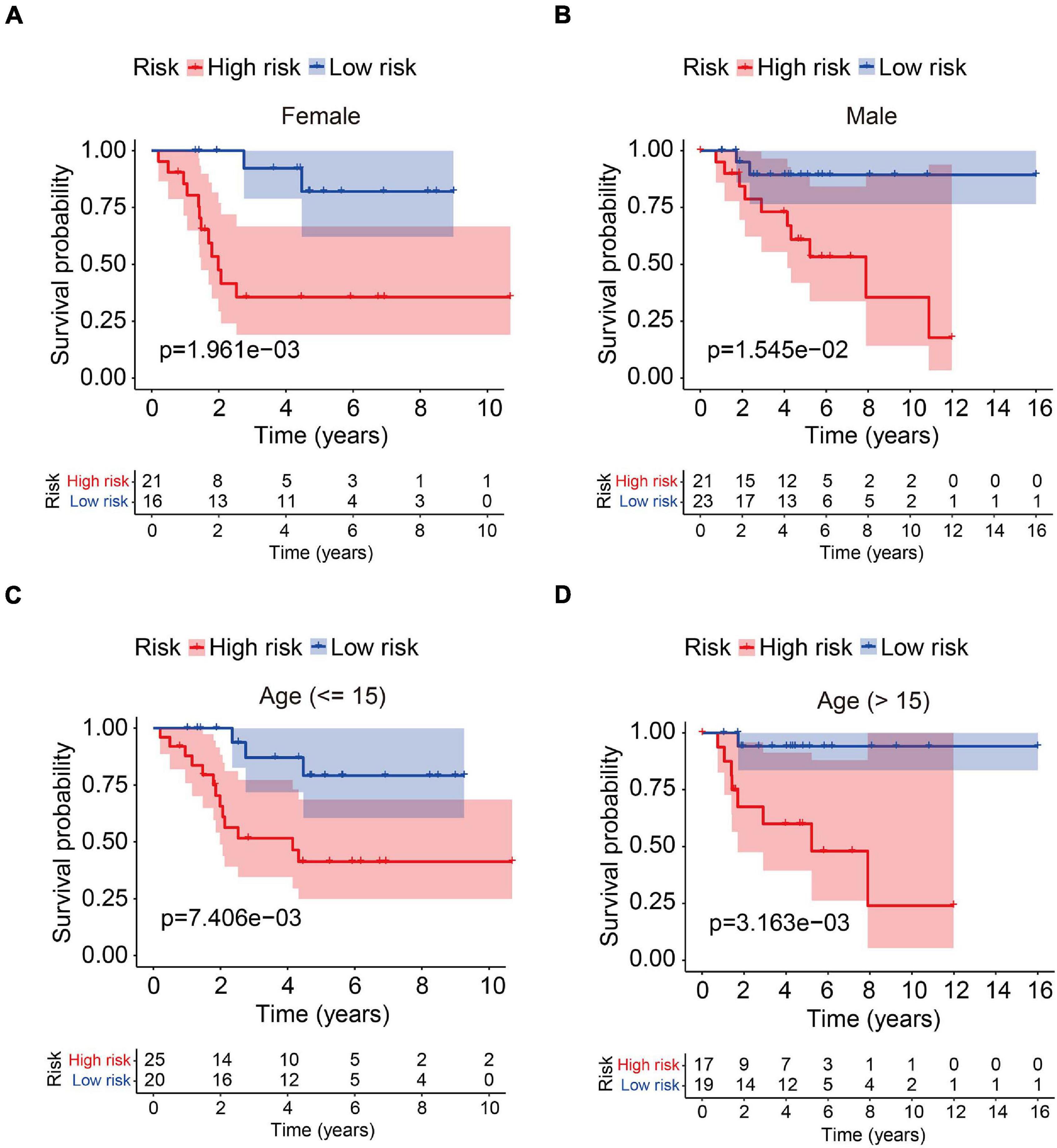
Figure 5. Analysis of the six-MRG signature in clinicopathological subtypes of osteosarcoma. (A,B) Kaplan-Meier survival curve analysis of high- and low-risk males and females. (C,D) Kaplan-Meier survival curve analysis of high- and low-risk patients aged ≤ 15 or age > 15 years.
Construction and Validation of a Nomogram in the TCGA Osteosarcoma Cohort
We also construct a nomogram based on the TCGA osteosarcoma cohort to facilitate clinical decision-making. Clinicopathological characteristics including age, gender, tumor site, and risk score were all included in the nomogram (Figure 6A). As shown in Figures 6B–D, the calibration curve suggested that the predicted 1-, 3-, and 5-year overall survival was consistent with the actual 1-, 3-, and 5-year overall survival.
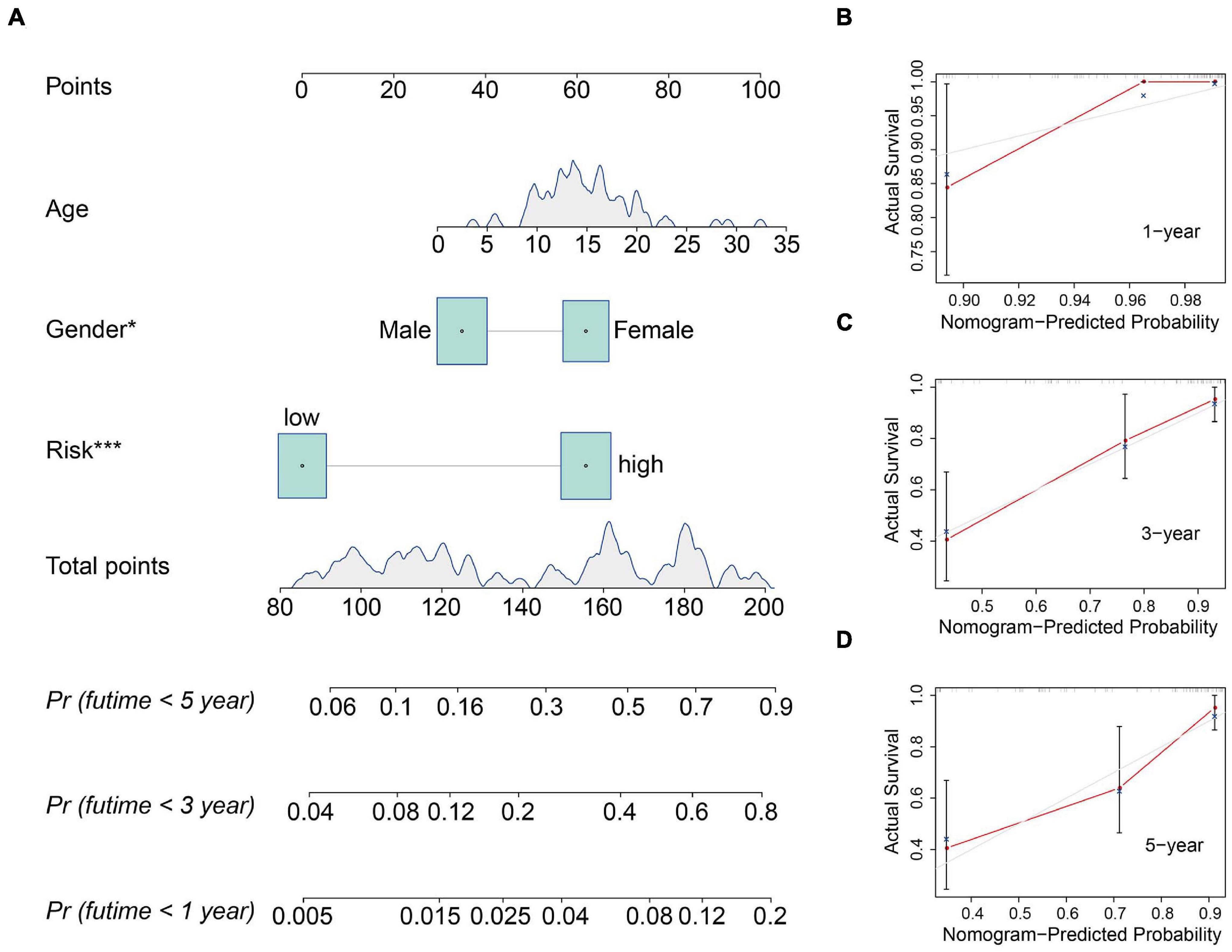
Figure 6. Construction and validation of a nomogram in the TCGA osteosarcoma cohort. (A) The nomogram consists of age, gender, and risk score based on the six-MRG signature. (B–D) The calibration curve for internal validation of the nomogram for estimating the survival of osteosarcoma patients at 1, 3, and 5 years.
Functional Analyses in the TCGA Osteosarcoma Cohort and GSE39055 Cohort
To further examine the biological processes and signaling pathways related to the risk score, we conducted functional enrichment analysis of genes differentially expressed between the high- and low-risk groups in the TCGA osteosarcoma and GSE39055 cohorts. GO enrichment analysis showed that cellular components including focal adhesion, cell-substrate adherens junction, cell-substrate junction, and collagen-containing extracellular matrix processes were significantly enriched in both the TCGA and GSE39055 cohorts (Figures 7A,B). Interestingly, KEGG pathway enrichment analysis also suggested that focal adhesion was one of the two pathways significantly enriched in the DEGs from the TCGA cohort and validated in the GSE39055 cohort, as was the Rap1 signaling pathway (Figures 7C,D). GSEA on the gene expression matrix of the TCGA cohort revealed that several cancer-related pathways including cell cycle and focal adhesion were significantly enriched in the high-risk group, and these enriched pathways were validated on the gene expression matrix of the GSE39055 cohort (Figures 7E,F).
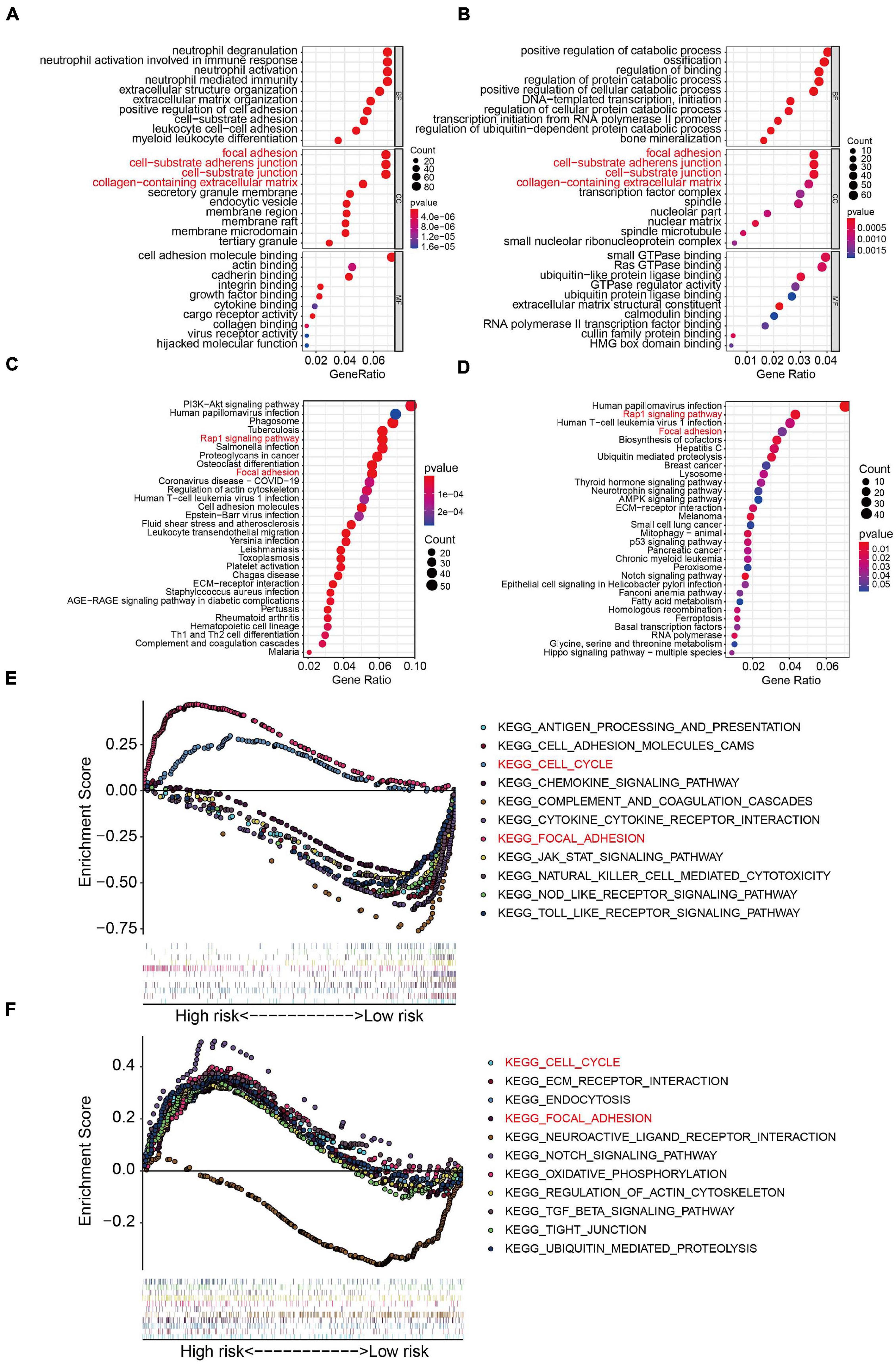
Figure 7. Functional analyses in the TCGA osteosarcoma dataset and GSE39055 dataset. (A–B) GO analysis of differentially expressed genes between the high- and low-risk groups in the TCGA osteosarcoma and GSE39055 datasets. (C–D) KEGG enrichment analysis of the differentially expressed genes in the TCGA osteosarcoma and GSE39055 datasets. (E,F) Gene set enrichment analysis in the TCGA osteosarcoma and GSE39055 datasets.
Differential Expression of the Six MRGs in Osteosarcoma
To elucidate the biological function of the six MRGs in osteosarcoma, we first analyzed their expression in primary and metastatic osteosarcoma samples in the GSE14359 and GSE32981 datasets. Expression of ABCA3, CTGF, and AMIGO2 were significantly higher in metastatic osteosarcoma samples compared to the primary osteosarcoma samples (Supplementary Figures 1A–C) while the expression of PREB, FHIT, and EXOSC5 were significantly decreased in metastatic osteosarcoma samples (Supplementary Figures 1D–F). Among the six MRGs, FHIT is a well-documented tumor suppressor and its expression was also downregulated in other malignant tumors including bladder, breast, esophageal cancer, head and neck squamous cell carcinoma, renal chromophobe cancers, renal clear cell carcinomas, lung squamous cell carcinomas, and thyroid carcinomas (Figure 8A). However, little is known about the role of FHIT in osteosarcoma. We therefore quantified the expression of FHIT in normal osteoblasts (hFOB1.19 cells) and osteosarcoma cells including 143B, U2OS, MG63, and HOS. qRT-PCR and western blotting showed that the mRNA and protein levels of FHIT were significantly lower in osteosarcoma cells compared to normal osteoblasts (Figures 8B,C). Consistently, FHIT mRNA and protein levels were significantly lower in osteosarcoma tissues than in adjacent normal tissues (Figures 8D–F).
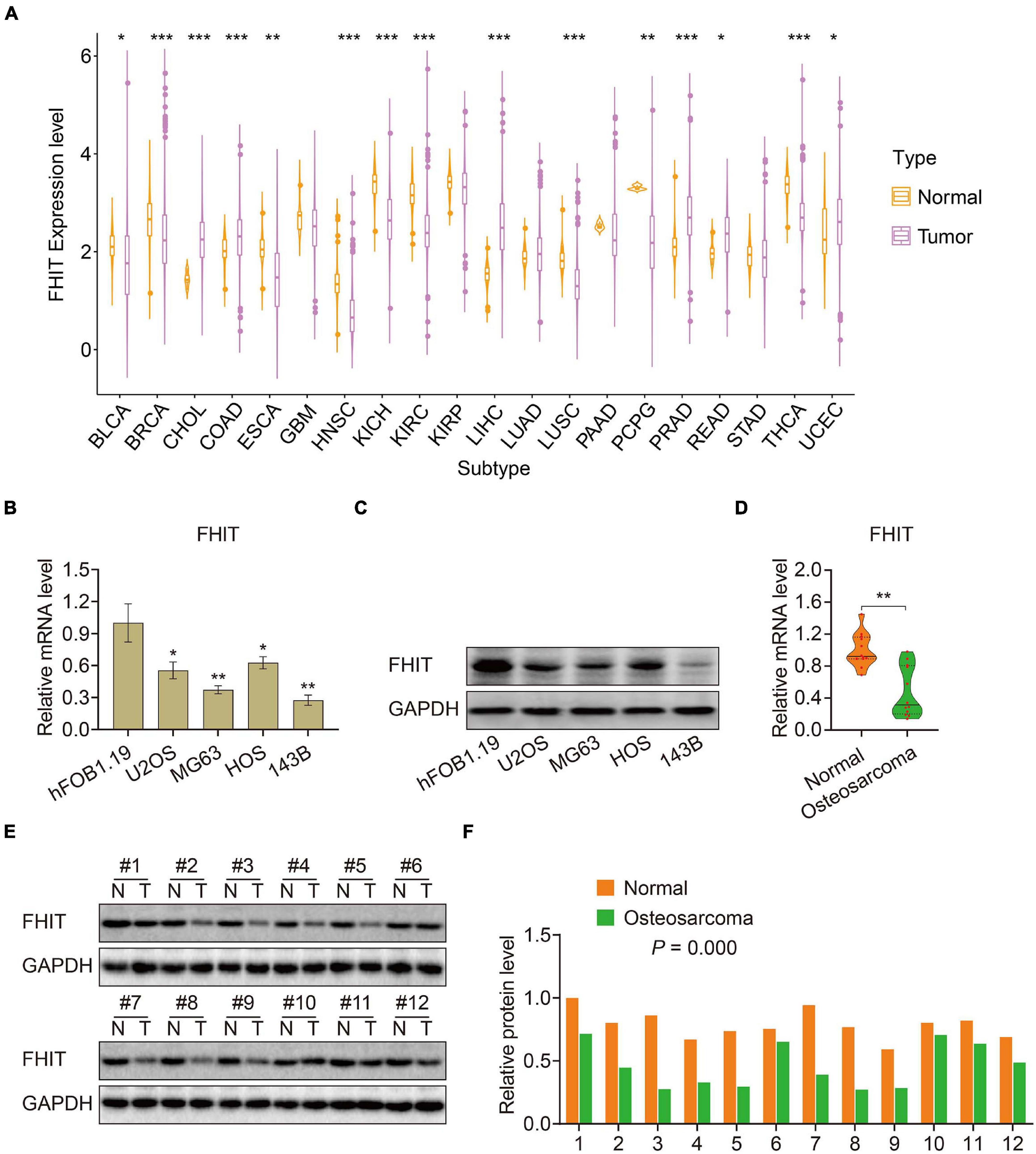
Figure 8. FHIT expression in osteosarcoma cells, tumor tissues, and other malignant tumors. (A) FHIT expression in other malignant tumors and adjacent normal tissues. (B,C) qRT-PCR and western blotting were performed to detect mRNA and protein expression of FHIT in osteosarcoma cells and normal osteoblasts. (D,E) mRNA and protein levels of FHIT were explored by qRT-PCR and western blotting. (F) Quantitative analysis of the western blot results. *P < 0.05, **P < 0.01, ***P < 0.001.
FHIT Suppresses Osteosarcoma Cell Proliferation and EMT
To explore the role of FHIT in osteosarcoma, we first upregulated FHIT expression in 143B and MG63 cells by lentivirus transfection (Supplementary Figure 2) and then detected the effect of FHIT overexpression on cellular proliferation. As shown in Figures 9A–D, the CCK-8 and colony formation assays suggested that FHIT overexpression significantly inhibited 143B and MG63 cell proliferation. Cell migration and invasion are closely associated with osteosarcoma metastasis, so we further examined the effect of FHIT overexpression on osteosarcoma cell migration and invasion. As shown in Figures 9E–I, the migration and invasion of 143B and MG63 cells were significantly inhibited by FHIT overexpression. To further explore the mechanism underlying the inhibitory effect of FHIT in osteosarcoma, we quantified the expression of EMT related-genes in FHIT-overexpressing osteosarcoma cells. As shown in Figures 9J–M, FHIT overexpression decreased the mRNA and protein expression of Ki67, N-cadherin, and vimentin but increased expression of E-cadherin in osteosarcoma cells.
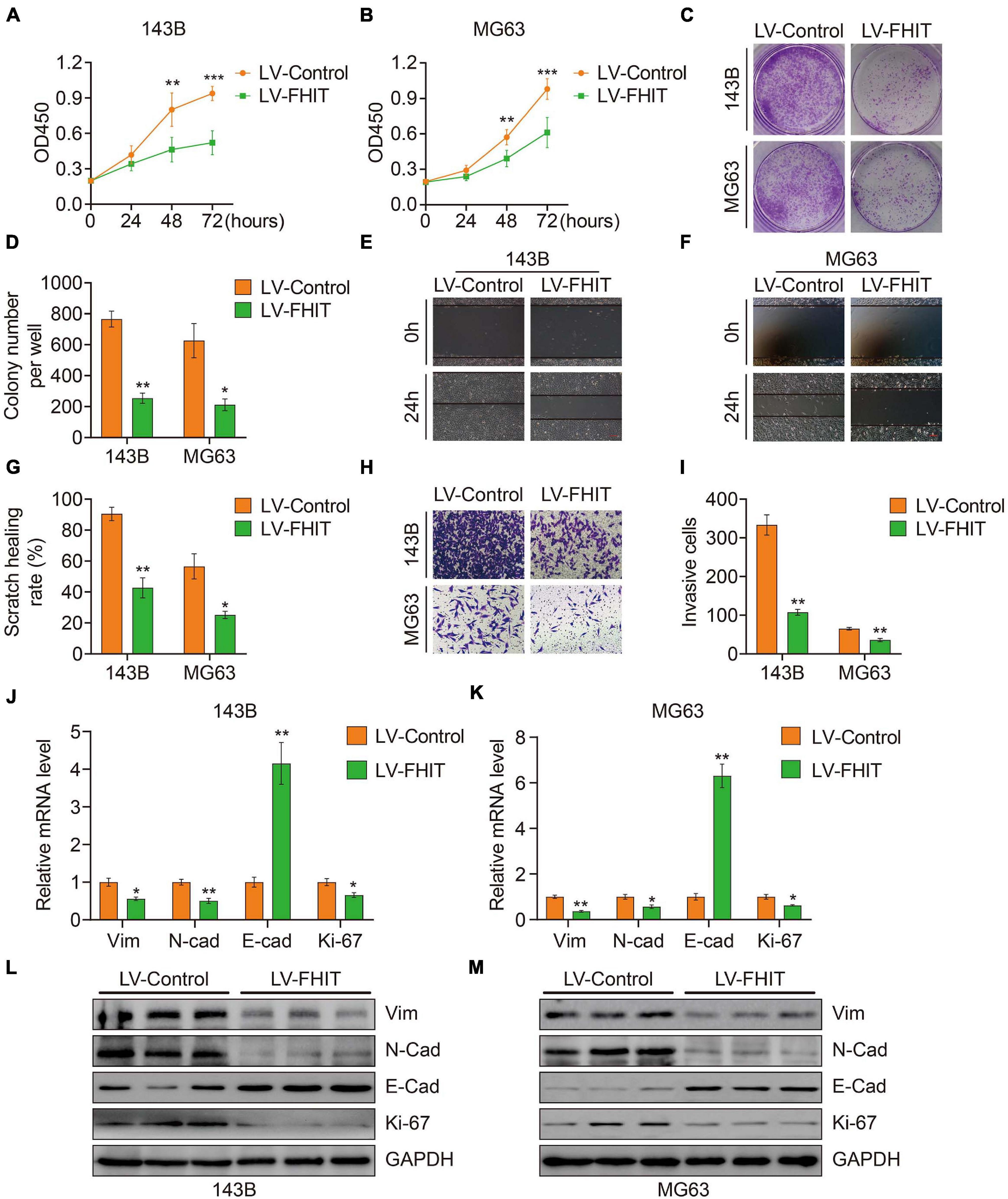
Figure 9. The effect of FHIT on osteosarcoma cell proliferation, migration, and invasion. CCK-8 (A,B) and colony formation assays (C,D) were used to assess the osteosarcoma cell proliferation. (E–G) A wound healing assay was performed to estimate the effect of FHIT overexpression on cell migration. Scale bar, 0.2 mm. (H,I) A transwell invasion assay was conducted to assess the effect of FHIT overexpression on osteosarcoma cell invasion. (J,K) The mRNA expression levels of EMT-related genes were detected by qRT-PCR. (L,M) Protein expression levels of vimentin, N-cadherin, E-cadherin, and Ki67 after overexpression of FHIT were measured by western blot analysis. *P < 0.05, **P < 0.01, ***P < 0.001.
FHIT Inhibits Tumor Growth in vivo
Finally, we explored the effect of FHIT overexpression on osteosarcoma growth in vivo. FHIT-overexpressing 143B cells and control cells were injected subcutaneously into athymic nude mice and tumor volumes and weights were monitored. FHIT overexpression, confirmed by qPCR (Figure 10D), resulted in a significant reduction in tumor volume and weight (Figures 10A–C). Furthermore, micro-metastases were detected in the lung tissues of nude mice injected with LV-Control 143B cells (Figure 10E) but not in mice injected with LV-FHIT 143B cells. Furthermore, analysis of the expression of EMT-related genes in subcutaneous tumors by western blot and immunohistochemistry showed that Ki67, N-cadherin, and vimentin were significantly lower in the LV-FHIT group than in the LV-Control group, while E-cadherin expression markedly increased (Figures 10F,G). Moreover, analysis of the correlation of FHIT with EMT-related genes in GSE16091 dataset showed that FHIT was positively correlated with E-cadherin, while negatively correlated with vimentin (Figures 10H,I). These results suggest that FHIT inhibits epithelial-mesenchymal transition in osteosarcoma.
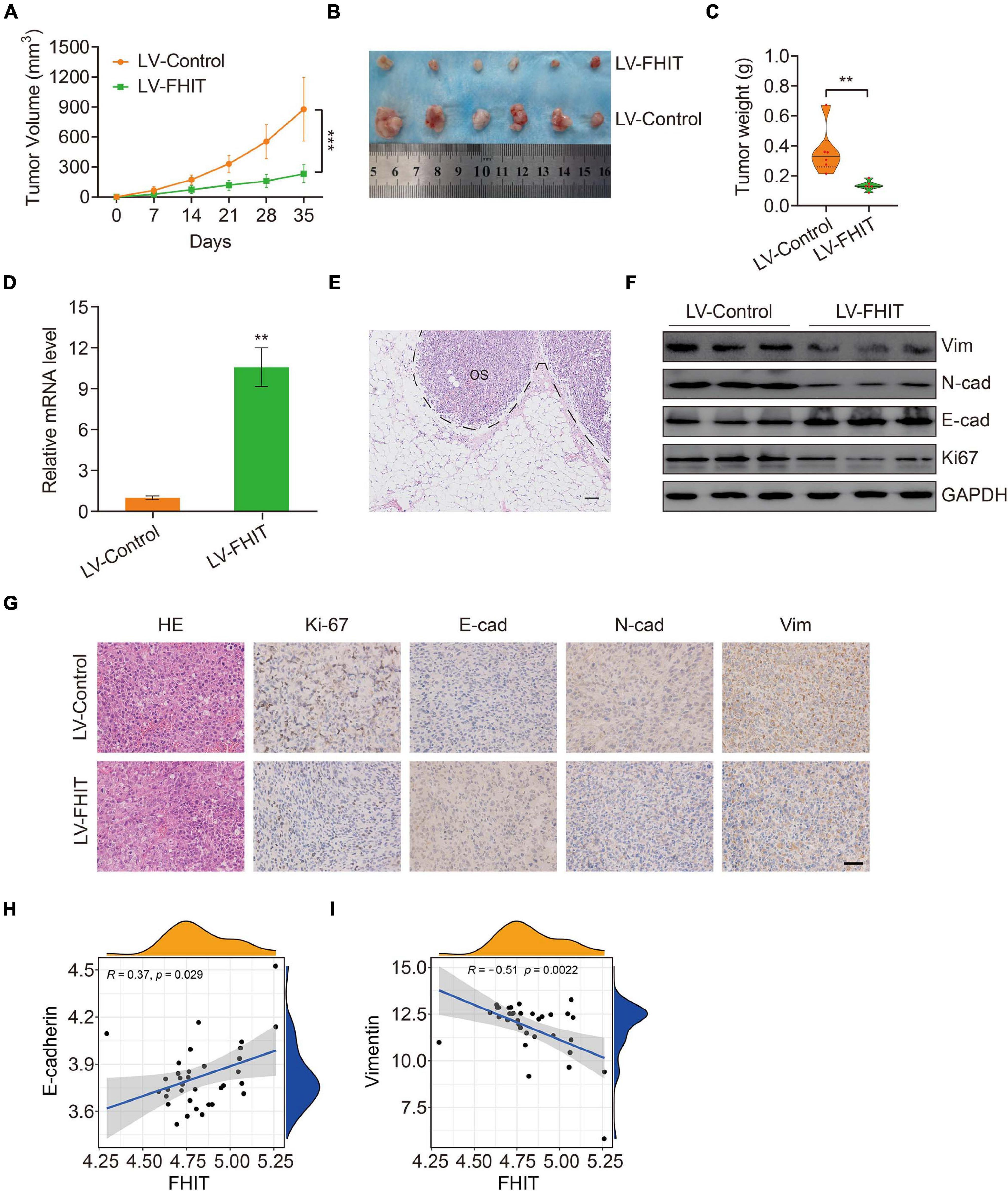
Figure 10. FHIT inhibits tumor growth in vivo. (A) Tumor volumes were monitored every week. (B) Subcutaneous tumors were dissected and photographed after 5 weeks. (C) Tumor weights are shown. (D) qRT-PCR was carried out to quantify the FHIT expression in subcutaneous tumor tissues. (E) Representative image of micro-metastasis in the lung tissues of nude mice injected with LV-Control 143B cells. OS, osteosarcoma. Scale bar, 1 cm. (F) Protein expression levels of vimentin, N-cadherin, E-cadherin, and Ki67 were measured by western blot analysis. (G) Immunohistochemistry analysis of Ki67, E-cadherin, N-cadherin, and vimentin in tumors. Scale bar, 0.5 cm. (H,I) The correlation of FHIT with E-cadherin and vimentin in GSE16091 dataset. **P < 0.01, ***P < 0.001.
Discussion
Metastasis is a typical hallmark of cancer and the main cause of osteosarcoma death (Hanahan and Weinberg, 2011; Moses et al., 2018). However, the mechanisms underlying osteosarcoma metastasis are largely unknown. Epithelial-mesenchymal transition (EMT) is a key process driving tumor metastasis (Mittal, 2018; Pastushenko and Blanpain, 2019). EMT is a dynamic and reversible process characterized by cells losing their epithelial-like features and transforming into cells with mesenchymal characteristics (Lu and Kang, 2019). Cells undergoing EMT have enhanced migratory capacity, contributing to their aggressive clinical behavior (Mittal, 2018). Though osteosarcoma cells are considered to arise from mesenchymal cells, recent studies have revealed that EMT occurs in osteosarcoma and contributes to its initiation, progression, and metastasis (Hou et al., 2014; Shen et al., 2017; Han et al., 2019; Xu et al., 2020). Many genes including EMT-related transcription factors and non-coding RNAs are associated with EMT and either promote or inhibit osteosarcoma metastasis. For example, the long non-coding (lnc)RNA AFAP1-AS1 acts as an oncogene to promote EMT and osteosarcoma metastasis. Mechanistically, AFAP1-AS1 could interact with RhoC, thereby activating the RhoC/ROCK1/p38MAPK/Twist1 signaling pathway (Shi et al., 2019). Plasma levels of miR-22 were also found to be an independent diagnostic and prognostic biomarker in osteosarcoma (Diao et al., 2020). Moreover, at the cellular level, miR-22 overexpression impaired proliferation and EMT in osteosarcoma by targeting Twist1 (Zhu et al., 2020). These studies suggest that EMT and distant metastasis are tightly regulated in osteosarcoma. However, the specific molecular events governing EMT and metastasis in osteosarcoma are still poorly understood.
Here we identified genes that were differentially expressed between primary and metastatic osteosarcoma, which we term MRGs. Functional analysis of the MRGs suggested that TNF and NF-kappa B signaling were significantly enriched in metastasis, two pathways that are known to regulate metastasis in a variety of tumors including osteosarcoma (Liao et al., 2015; Ren et al., 2017; Liu et al., 2020). We constructed a prognostic model for osteosarcoma patients comprising six MRGs that divided patients in both internal and external validation cohorts into high- and low-risk groups according to a median risk derived from patients in a training cohort. Kaplan-Meier survival analysis suggested that patients in the high-risk group had poor overall survival in the training cohort and all validation cohorts. To further evaluate the prognostic value of the model, Kaplan-Meier survival analysis was performed in various clinical sub-groups in the TCGA cohort. Consistently, in all clinical sub-groups, patients in the high-risk group had poorer overall survival than those in the low-risk group. ROC curve analysis confirmed the satisfactory accuracy of the prognostic model. Moreover, univariate and multivariate Cox regression analyses suggested that the risk score was independently prognostic in osteosarcoma patients. Taken together, these results suggest that the six-MRG signature had a robust prognostic value in osteosarcoma patients and was generalizable to other cohorts. A nomogram that included clinicopathological characteristics including age, gender, tumor site, and risk score was also designed to facilitate clinical decision-making.
To elucidate the molecular mechanism underlying osteosarcoma metastasis, we first identified those genes that were differentially expressed between high- and low-risk patients in the TCGA and GSE39055 cohorts. Functional enrichment analyses revealed that DEGs from both cohorts were significantly enriched in focal adhesion, suggesting a potential role in osteosarcoma metastasis. There is increasing evidence that focal adhesion is a vital determinant of cell migration and a promoter of tumor invasion and metastasis (Eke and Cordes, 2015; Shen et al., 2018). Thus, our analysis connected focal adhesion with osteosarcoma metastasis, a mechanism that requires further exploration.
The six MRGs used in the prognostic model were ABCA3, CTGF, AMIGO2, PREB, EXOSC5, and FHIT. The expression of the first three of these was increased in metastatic compared with primary osteosarcomas, while the latter three were decreased, suggesting differential functions for these genes in the metastatic process. ABCA3 is a highly conserved multi-membrane-spanning protein belonging to the ATP-binding cassette transporter superfamily and that plays a critical role in maintaining pulmonary surfactant homeostasis, with mutations confirmed to contribute to lung diseases including lung cancer (Beers and Mulugeta, 2017). Steinbach et al. (2006) established a link between aberrant ABCA3 expression and multi-drug resistance in childhood acute myeloid leukemia, suggesting a potential role for ABCA3 in malignant tumors. However, until now, ABCA3 has not been implicated in tumor metastasis.
Connective tissue growth factor (CTGF) is a multifunctional signaling modulator that is increasingly recognized as promoting tumor metastasis by regulating cellular proliferation, migration, and invasion (Shen et al., 2020). Wang et al. (2017) recently reported that CTGF might promote osteosarcoma angiogenesis and metastasis by upregulating expression of Angpt2, consistent with our finding that CTGF is upregulated in metastatic osteosarcoma and is pro-metastatic. AMIGO2 (adhesion molecule with Ig-like domain 2) is a transmembrane protein with known oncoprotein function that is described as a driver of metastasis in breast and ovarian cancer and metastatic melanoma (Fontanals-Cirera et al., 2017; Sonzogni et al., 2018; Liu et al., 2021). The prolactin regulatory element binding (PREB) gene encodes a transcription factor that regulates target genes by binding to the prolactin gene (PRL) promoter (Zhang et al., 2017). PREB has described functions in hepatic glucose homeostasis (Park et al., 2018) and hepatitis C virus replication (Kong et al., 2016) but, to date, it is uncharacterized in cancer. Exosome component 5 (EXOSC5), also known as CML28 or Rrp46p, is an RNA exosome complex subunit overexpressed in various epithelial and hematopoietic tumor cell lines (Yang et al., 2002; Wu et al., 2005). In epithelial tumors such as colorectal cancer, EXOSC5 overexpression promotes proliferation by activating the ERK and AKT pathways (Pan et al., 2019), but its expression and role in mesenchymal tumors are unknown. Contrary to these previously described oncogenic functions, we found that EXOSC5 was downregulated in metastatic osteosarcoma so might have a tumor suppressor function in this tissue context.
The fragile histidine triad (FHIT) gene (located at 3p14.2) is a bona fide tumor suppressor. Hypermethylation of FHIT is commonly detected in human cancers, resulting in its inactivation as a very early event in cancer formation (Foja et al., 2005; Yan et al., 2016). Abnormal methylation or aberrant expression of FHIT has been shown to influence tumor cell proliferation, migration, invasion, and metastasis in a variety of cancers (Vecchione et al., 2004; Suh et al., 2014). FHIT expression is reduced in many breast cancers, and its nuclear translocation promotes breast cancer cell proliferation (Bianchi et al., 2015); moreover, loss of FHIT expression or function were correlated with patient poor prognosis and advanced stage (Campiglio et al., 1999; Arun et al., 2005). In lung cancer, FHIT hypermethylation, which inactivated FHIT, was significantly higher in tumors than in normal lung tissues. FHIT hypermethylation was also associated with an increased risk of lung cancer and a worse overall survival from the disease (Yan et al., 2016). Re-expression of FHIT in FHIT-negative highly invasive lung cancer cells inhibited migration and invasion, while FHIT knockdown in FHIT-positive poorly invasive cells increased their migratory or invasive potential (Joannes et al., 2010). This evidence indicates a critical role for FHIT in tumor metastasis. In osteosarcoma, Hinohara et al. (1998) reported abnormalities in FHIT transcripts but no functional analysis was performed. Xu et al. (2019) reported that FHIT expression was lower in osteosarcoma cells than that in normal osteoblasts and that its overexpression inhibited proliferation and promoted apoptosis of osteosarcoma cells. We found that FHIT overexpression reduced the migratory and invasive capacities of osteosarcoma cells. Mechanistically, FHIT overexpression repressed N-cadherin, vimentin, and Ki67 and increased the expression of E-cadherin, suggesting that its overexpression inhibits EMT in osteosarcoma cells. Our results suggest a metastasis-inhibiting potential for FHIT in osteosarcoma, making it a therapeutic target worthy of further investigation.
This study has some limitations. First, our MRGs were identified according to their differential expression between primary and metastatic osteosarcoma, which may have excluded some functional genes that were not differentially expressed in those tissues or at a different point in time. The detailed mechanism underlying how FHIT inhibits EMT also requires further exploration.
Nevertheless, we identified a novel six-MRG prognostic signature for osteosarcoma patients and a derived risk score that was a robust independent prognostic biomarker. Moreover, we constructed a nomogram to aid clinical decision-making and to personalize treatment for osteosarcoma patients. Of the six MRGs, FHIT is a potent tumor suppressor and its overexpression inhibited the migration, invasion, and metastasis of osteosarcoma by inhibiting EMT. FHIT therefore represents a promising therapeutic target in osteosarcoma patients.
Data Availability Statement
Publicly available datasets were analyzed in this study. The names of the repository/repositories and accession number(s) can be found in the article/Supplementary Material.
Ethics Statement
The studies involving human participants were reviewed and approved by the Ethics Committee of Wuhan University People’s Hospital. The patients/participants provided their written informed consent to participate in this study. The animal study was reviewed and approved by Ethics Committee of Wuhan University People’s Hospital.
Author Contributions
WG and JY designed the study. KX, CG, and YQ completed the experiments. YS and WL analyzed the data. DZ conducted bioinformatic analysis, wrote the manuscript, and was responsible for language revisions. All authors reviewed the manuscript.
Funding
This study was funded by grants from Science and Technology Major Project of Hubei Province (2020BCB058 and 2019CFB175) and National Natural Science Foundation of China (81341078).
Conflict of Interest
The authors declare that the research was conducted in the absence of any commercial or financial relationships that could be construed as a potential conflict of interest.
Publisher’s Note
All claims expressed in this article are solely those of the authors and do not necessarily represent those of their affiliated organizations, or those of the publisher, the editors and the reviewers. Any product that may be evaluated in this article, or claim that may be made by its manufacturer, is not guaranteed or endorsed by the publisher.
Acknowledgments
We sincerely thank the TCGA project for using their data.
Supplementary Material
The Supplementary Material for this article can be found online at: https://www.frontiersin.org/articles/10.3389/fcell.2021.699212/full#supplementary-material
Footnotes
References
Arun, B., Kilic, G., Yen, C., Foster, B., Yardley, D. A., Gaynor, R., et al. (2005). Loss of FHIT expression in breast cancer is correlated with poor prognostic markers. Cancer Epidemiol. Biomarkers Prev. 14, 1681–1685. doi: 10.1158/1055-9965.epi-04-0278
Beers, M. F., and Mulugeta, S. (2017). The biology of the ABCA3 lipid transporter in lung health and disease. Cell Tissue Res. 367, 481–493. doi: 10.1007/s00441-016-2554-z
Bianchi, F., Sasso, M., Turdo, F., Beretta, G. L., Casalini, P., Ghirelli, C., et al. (2015). Fhit nuclear import following EGF stimulation sustains proliferation of breast cancer cells. J. Cell. Physiol. 230, 2661–2670. doi: 10.1002/jcp.24968
Campiglio, M., Pekarsky, Y., Menard, S., Tagliabue, E., Pilotti, S., and Croce, C. M. (1999). FHIT loss of function in human primary breast cancer correlates with advanced stage of the disease. Cancer Res. 59, 3866–3869.
Deng, Q., Li, P., Che, M., Liu, J., Biswas, S., Ma, G., et al. (2019). Activation of hedgehog signaling in mesenchymal stem cells induces cartilage and bone tumor formation via Wnt/beta-Catenin. Elife 8:e50208.
Diao, Z. B., Sun, T. X., Zong, Y., Lin, B. C., and Xia, Y. S. (2020). Identification of plasma microRNA-22 as a marker for the diagnosis, prognosis, and chemosensitivity prediction of osteosarcoma. J. Int. Med. Res. 48:300060520967818.
Eke, I., and Cordes, N. (2015). Focal adhesion signaling and therapy resistance in cancer. Semin. Cancer Biol. 31, 65–75. doi: 10.1016/j.semcancer.2014.07.009
Foja, S., Goldberg, M., Schagdarsurengin, U., Dammann, R., Tannapfel, A., and Ballhausen, W. G. (2005). Promoter methylation and loss of coding exons of the fragile histidine triad (FHIT) gene in intrahepatic cholangiocarcinomas. Liver Int. 25, 1202–1208. doi: 10.1111/j.1478-3231.2005.01174.x
Fontanals-Cirera, B., Hasson, D., Vardabasso, C., Di Micco, R., Agrawal, P., Chowdhury, A., et al. (2017). Harnessing BET inhibitor sensitivity reveals AMIGO2 as a melanoma survival gene. Mol. Cell 68, 731–744.e9.
Gianferante, D. M., Mirabello, L., and Savage, S. A. (2017). Germline and somatic genetics of osteosarcoma–connecting aetiology, biology and therapy. Nat. Rev. Endocrinol. 13, 480–491. doi: 10.1038/nrendo.2017.16
Han, Y., Guo, W., Ren, T., Huang, Y., Wang, S., Liu, K., et al. (2019). Tumor-associated macrophages promote lung metastasis and induce epithelial-mesenchymal transition in osteosarcoma by activating the COX-2/STAT3 axis. Cancer Lett. 440-441, 116–125. doi: 10.1016/j.canlet.2018.10.011
Hanahan, D., and Weinberg, R. A. (2011). Hallmarks of cancer: the next generation. Cell 144, 646–674. doi: 10.1016/j.cell.2011.02.013
Harrison, D. J., Geller, D. S., Gill, J. D., Lewis, V. O., and Gorlick, R. (2018). Current and future therapeutic approaches for osteosarcoma. Expert Rev. Anticancer Ther. 18, 39–50.
Hinohara, S., Satake, N., Sekine, K., and Kaneko, Y. (1998). Abnormalities of the FHIT transcripts in osteosarcoma and Ewing sarcoma. Jpn. J. Cancer Res. 89, 887–894. doi: 10.1111/j.1349-7006.1998.tb00645.x
Hou, C. H., Lin, F. L., Hou, S. M., and Liu, J. F. (2014). Cyr61 promotes epithelial-mesenchymal transition and tumor metastasis of osteosarcoma by Raf-1/MEK/ERK/Elk-1/TWIST-1 signaling pathway. Mol. Cancer 13:236. doi: 10.1186/1476-4598-13-236
Joannes, A., Bonnomet, A., Bindels, S., Polette, M., Gilles, C., Burlet, H., et al. (2010). Fhit regulates invasion of lung tumor cells. Oncogene 29, 1203–1213. doi: 10.1038/onc.2009.418
Kansara, M., Teng, M. W., Smyth, M. J., and Thomas, D. M. (2014). Translational biology of osteosarcoma. Nat. Rev. Cancer 14, 722–735.
Kong, L., Fujimoto, A., Nakamura, M., Aoyagi, H., Matsuda, M., Watashi, K., et al. (2016). Prolactin regulatory element binding protein is involved in hepatitis C virus replication by interaction with NS4B. J. Virol. 90, 3093–3111. doi: 10.1128/jvi.01540-15
Liao, D., Zhong, L., Duan, T., Zhang, R. H., Wang, X., Wang, G., et al. (2015). Aspirin suppresses the growth and metastasis of osteosarcoma through the NF-kappaB pathway. Clin. Cancer Res. 21, 5349–5359. doi: 10.1158/1078-0432.ccr-15-0198
Lin, Y. H., Jewell, B. E., Gingold, J., Lu, L., Zhao, R., Wang, L. L., et al. (2017). Osteosarcoma: molecular pathogenesis and iPSC modeling. Trends Mol. Med. 23, 737–755. doi: 10.1016/j.molmed.2017.06.004
Liu, J., Wu, Z., Han, D., Wei, C., Liang, Y., Jiang, T., et al. (2020). Mesencephalic astrocyte-derived neurotrophic factor inhibits liver cancer through small ubiquitin-related odifier (SUMO)ylation-related suppression of NF-kappaB/Snail signaling pathway and epithelial-mesenchymal transition. Hepatology 71, 1262–1278. doi: 10.1002/hep.30917
Liu, S., Liu, J., Yu, X., Shen, T., and Fu, Q. (2019). Identification of a two-gene (PML-EPB41) signature with independent prognostic value in osteosarcoma. Front. Oncol. 9:1578. doi: 10.3389/fonc.2019.01578
Liu, Y., Yang, J., Shi, Z., Tan, X., Jin, N., O’brien, C., et al. (2021). In vivo selection of highly metastatic human ovarian cancer sublines reveals role for AMIGO2 in intra-peritoneal metastatic regulation. Cancer Lett. 503, 163–173. doi: 10.1016/j.canlet.2021.01.024
Lu, W., and Kang, Y. (2019). Epithelial-mesenchymal plasticity in cancer progression and metastasis. Dev. Cell 49, 361–374. doi: 10.1016/j.devcel.2019.04.010
Marchandet, L., Lallier, M., Charrier, C., Baud’huin, M., Ory, B., and Lamoureux, F. (2021). Mechanisms of resistance to conventional therapies for osteosarcoma. Cancers 13:683. doi: 10.3390/cancers13040683
Mittal, V. (2018). Epithelial mesenchymal transition in tumor metastasis. Annu. Rev. Pathol. 13, 395–412.
Moses, C., Garcia-Bloj, B., Harvey, A. R., and Blancafort, P. (2018). Hallmarks of cancer: the CRISPR generation. Eur. J. Cancer 93, 10–18. doi: 10.1016/j.ejca.2018.01.002
Pan, H., Pan, J., Song, S., Ji, L., Lv, H., and Yang, Z. (2019). EXOSC5 as a novel prognostic marker promotes proliferation of colorectal cancer via activating the ERK and AKT pathways. Front. Oncol. 9:643. doi: 10.3389/fonc.2019.00643
Park, J. M., Kim, M. Y., Kim, T. H., Min, D. K., Yang, G. E., and Ahn, Y. H. (2018). Prolactin regulatory element-binding (PREB) protein regulates hepatic glucose homeostasis. Biochim. Biophys. Acta Mol. Basis Dis. 1864(6 Pt A), 2097–2107. doi: 10.1016/j.bbadis.2018.03.024
Pastushenko, I., and Blanpain, C. (2019). EMT transition states during tumor progression and metastasis. Trends Cell Biol. 29, 212–226. doi: 10.1016/j.tcb.2018.12.001
Prudowsky, Z. D., and Yustein, J. T. (2020). Recent insights into therapy resistance in osteosarcoma. Cancers 13:83. doi: 10.3390/cancers13010083
Ren, D., Yang, Q., Dai, Y., Guo, W., Du, H., Song, L., et al. (2017). Oncogenic miR-210-3p promotes prostate cancer cell EMT and bone metastasis via NF-kappaB signaling pathway. Mol. Cancer 16:117.
Rickel, K., Fang, F., and Tao, J. (2017). Molecular genetics of osteosarcoma. Bone 102, 69–79. doi: 10.1016/j.bone.2016.10.017
Sayles, L. C., Breese, M. R., Koehne, A. L., Leung, S. G., Lee, A. G., Liu, H. Y., et al. (2019). Genome-informed targeted therapy for osteosarcoma. Cancer Discov. 9, 46–63.
Shen, J., Cao, B., Wang, Y., Ma, C., Zeng, Z., Liu, L., et al. (2018). Hippo component YAP promotes focal adhesion and tumour aggressiveness via transcriptionally activating THBS1/FAK signalling in breast cancer. J. Exp. Clin. Cancer Res. 37:175.
Shen, S., Huang, K., Wu, Y., Ma, Y., Wang, J., Qin, F., et al. (2017). A miR-135b-TAZ positive feedback loop promotes epithelial-mesenchymal transition (EMT) and tumorigenesis in osteosarcoma. Cancer Lett. 407, 32–44. doi: 10.1016/j.canlet.2017.08.005
Shen, Y. W., Zhou, Y. D., Chen, H. Z., Luan, X., and Zhang, W. D. (2020). Targeting CTGF in cancer: an emerging therapeutic opportunity. Trends Cancer 7, 511–524. doi: 10.1016/j.trecan.2020.12.001
Shi, D., Wu, F., Mu, S., Hu, B., Zhong, B., Gao, F., et al. (2019). LncRNA AFAP1-AS1 promotes tumorigenesis and epithelial-mesenchymal transition of osteosarcoma through RhoC/ROCK1/p38MAPK/Twist1 signaling pathway. J. Exp. Clin. Cancer Res. 38:375.
Sonzogni, O., Haynes, J., Seifried, L. A., Kamel, Y. M., Huang, K., Begora, M. D., et al. (2018). Reporters to mark and eliminate basal or luminal epithelial cells in culture and in vivo. PLoS Biol. 16:e2004049. doi: 10.1371/journal.pbio.2004049
Steinbach, D., Gillet, J. P., Sauerbrey, A., Gruhn, B., Dawczynski, K., Bertholet, V., et al. (2006). ABCA3 as a possible cause of drug resistance in childhood acute myeloid leukemia. Clin. Cancer Res. 12(14 Pt 1), 4357–4363. doi: 10.1158/1078-0432.ccr-05-2587
Suh, S. S., Yoo, J. Y., Cui, R., Kaur, B., Huebner, K., Lee, T. K., et al. (2014). FHIT suppresses epithelial-mesenchymal transition (EMT) and metastasis in lung cancer through modulation of microRNAs. PLoS Genet. 10:e1004652. doi: 10.1371/journal.pgen.1004652
Vecchione, A., Sevignani, C., Giarnieri, E., Zanesi, N., Ishii, H., Cesari, R., et al. (2004). Inactivation of the FHIT gene favors bladder cancer development. Clin. Cancer Res. 10, 7607–7612. doi: 10.1158/1078-0432.ccr-04-0341
Wang, L. H., Tsai, H. C., Cheng, Y. C., Lin, C. Y., Huang, Y. L., Tsai, C. H., et al. (2017). CTGF promotes osteosarcoma angiogenesis by regulating miR-543/angiopoietin 2 signaling. Cancer Lett. 391, 28–37. doi: 10.1016/j.canlet.2017.01.013
Whelan, J. S., and Davis, L. E. (2018). Osteosarcoma, chondrosarcoma, and chordoma. J. Clin. Oncol. 36, 188–193. doi: 10.1200/jco.2017.75.1743
Wu, C. J., Biernacki, M., Kutok, J. L., Rogers, S., Chen, L., Yang, X. F., et al. (2005). Graft-versus-leukemia target antigens in chronic myelogenous leukemia are expressed on myeloid progenitor cells. Clin. Cancer Res. 11, 4504–4511. doi: 10.1158/1078-0432.ccr-05-0036
Wu, W., Jing, D., Meng, Z., Hu, B., Zhong, B., Deng, X., et al. (2020). FGD1 promotes tumor progression and regulates tumor immune response in osteosarcoma via inhibiting PTEN activity. Theranostics 10, 2859–2871. doi: 10.7150/thno.41279
Xu, W. N., Yang, R. Z., Zheng, H. L., Jiang, L. S., and Jiang, S. D. (2020). NDUFA4L2 regulated by HIF-1alpha promotes metastasis and epithelial-mesenchymal transition of osteosarcoma cells through inhibiting ROS production. Front. Cell Dev. Biol. 8:515051. doi: 10.3389/fcell.2020.515051
Xu, Z., Wu, J., Cai, P., Zhou, X., Yi, C., and Wang, B. (2019). Effects of FHIT gene on proliferation and apoptosis of osteosarcoma cells. Oncol. Lett. 17, 877–882.
Yan, W., Xu, N., Han, X., Zhou, X. M., and He, B. (2016). The clinicopathological significance of FHIT hypermethylation in non-small cell lung cancer, a meta-analysis and literature review. Sci. Rep. 6:19303.
Yang, X. F., Wu, C. J., Chen, L., Alyea, E. P., Canning, C., Kantoff, P., et al. (2002). CML28 is a broadly immunogenic antigen, which is overexpressed in tumor cells. Cancer Res. 62, 5517–5522.
Zhang, X. Z., Imachi, H., Lyu, J. Y., Fukunaga, K., Sato, S., Ibata, T., et al. (2017). Prolactin regulatory element-binding protein is involved in suppression of the adiponectin gene in vivo. J. Endocrinol. Invest. 40, 437–445. doi: 10.1007/s40618-016-0589-3
Zhao, J., Dean, D. C., Hornicek, F. J., Yu, X., and Duan, Z. (2020). Emerging next-generation sequencing-based discoveries for targeted osteosarcoma therapy. Cancer Lett. 474, 158–167. doi: 10.1016/j.canlet.2020.01.020
Zhou, X., Fan, Y., Ye, W., Jia, B., Yang, Y., and Liu, Y. (2020). Identification of the novel target genes for osteosarcoma therapy based on comprehensive bioinformatic analysis. DNA Cell Biol. 39, 1172–1180. doi: 10.1089/dna.2020.5377
Keywords: epithelial to mesenchymal transition, FHIT, metastasis, osteosarcoma, prognostic model
Citation: Zheng D, Xia K, Yu L, Gong C, Shi Y, Li W, Qiu Y, Yang J and Guo W (2021) A Novel Six Metastasis-Related Prognostic Gene Signature for Patients With Osteosarcoma. Front. Cell Dev. Biol. 9:699212. doi: 10.3389/fcell.2021.699212
Received: 23 April 2021; Accepted: 02 July 2021;
Published: 23 July 2021.
Edited by:
Claudia Tanja Mierke, Leipzig University, GermanyReviewed by:
Orest William Blaschuk, McGill University, CanadaWeiren Luo, The Second Affiliated Hospital of Southern University of Science and Technology, China
Patcharee Jearanaikoon, Khon Kaen University, Thailand
Copyright © 2021 Zheng, Xia, Yu, Gong, Shi, Li, Qiu, Yang and Guo. This is an open-access article distributed under the terms of the Creative Commons Attribution License (CC BY). The use, distribution or reproduction in other forums is permitted, provided the original author(s) and the copyright owner(s) are credited and that the original publication in this journal is cited, in accordance with accepted academic practice. No use, distribution or reproduction is permitted which does not comply with these terms.
*Correspondence: Jian Yang, yangjian1986@whu.edu.cn; Weichun Guo, guoweichun@aliyun.com
†These authors have contributed equally to this work and share first authorship